Unlocking Business Potential with Data Labeling in Machine Learning
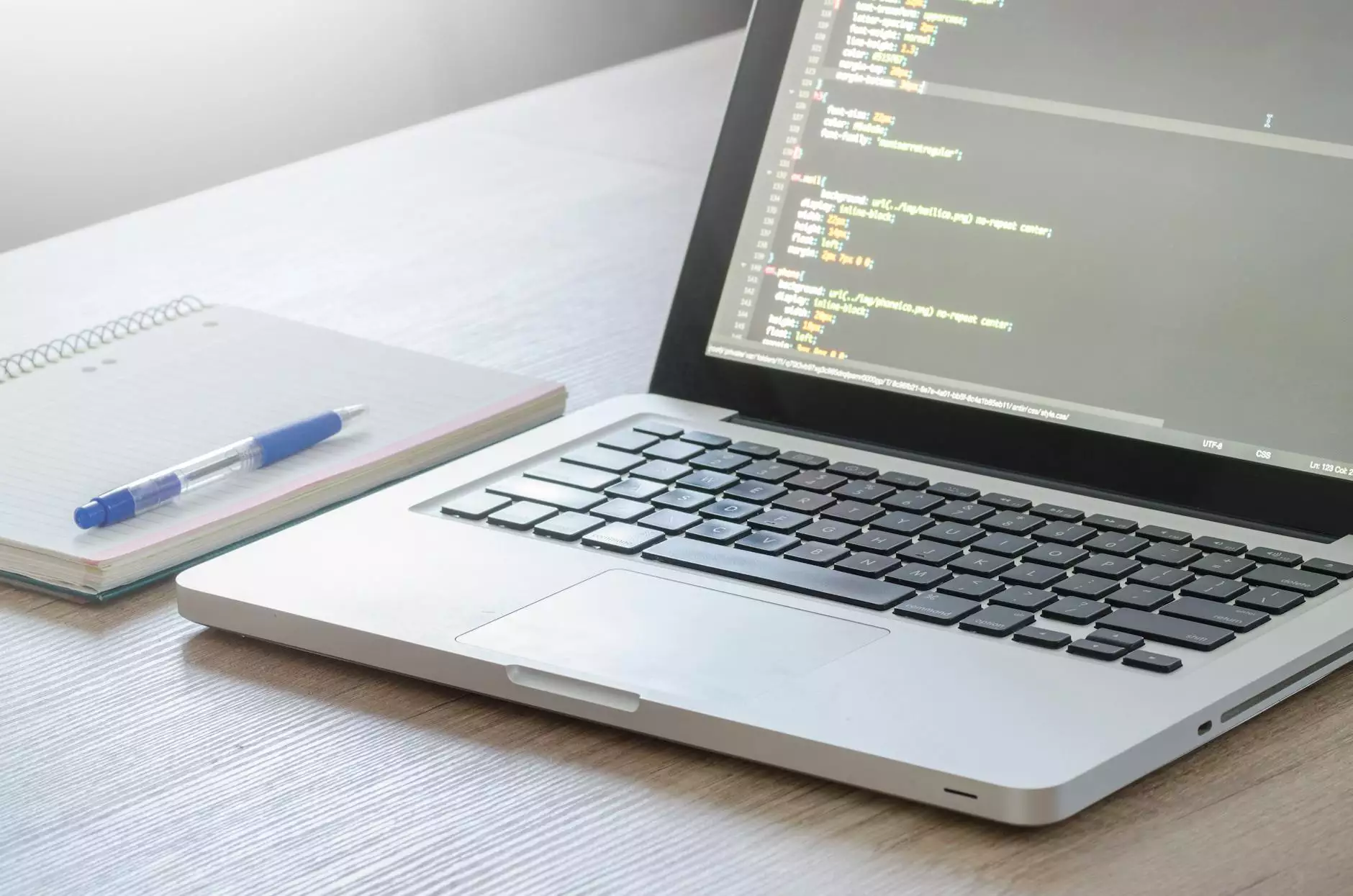
Understanding Data Labeling
Data labeling is the cornerstone of the machine learning process. It involves annotating datasets with labels that indicate the categorization or significance of the data. For instance, in image recognition, labeling involves identifying objects within an image, such as cars, pedestrians, and traffic signs. This annotated data is critical for training algorithms to recognize patterns and make predictions.
Businesses that harness the power of machine learning understand that data labeling is essential for developing accurate and efficient models. As the demand for precise machine learning applications rises, so does the need for high-quality data annotation tools and platforms.
The Importance of Data Annotation in Machine Learning
Data annotation lays the groundwork for machine learning algorithms to learn and improve over time. Here are a few reasons why it is crucial:
- Accuracy: Well-labeled data leads to more accurate models.
- Efficiency: Proper data annotation can reduce model training time significantly.
- Scalability: Annotated data allows for easily scalable machine learning applications.
- Comprehensiveness: High-quality data ensures that the learning algorithms can make well-informed decisions.
As businesses increasingly rely on data-driven decisions, understanding the importance of data labeling in machine learning becomes paramount for sustaining competitive advantages.
Key Labs: Pioneering Data Annotation Tools and Platforms
Key Labs is at the forefront of providing cutting-edge data annotation tools and platforms designed specifically for businesses looking to excel in the machine learning sphere. By utilizing Key Labs’ extensive suite of data labeling solutions, companies can accelerate their machine learning initiatives.
1. Comprehensive Data Annotation Tool
The Data Annotation Tool offered by Key Labs is equipped with advanced features that simplify the labeling process. Its intuitive interface has been designed to cater to both technical and non-technical users, which streamlines the workflow and improves productivity.
2. Robust Data Annotation Platform
The Data Annotation Platform from Key Labs allows businesses to manage large volumes of data efficiently. This platform leverages automation and human-in-the-loop capabilities to ensure high-quality labels while optimizing time and resources. Here are several key features:
- Scalable Solutions: Handle thousands of datasets with ease.
- Flexible Annotation Types: Supports various annotation methods, including image segmentation, keypoint annotation, and text classification.
- Quality Assurance: Implements a robust quality control system to ensure data integrity.
- Team Collaboration: Facilitates easy collaboration among team members, ensuring seamless project management.
By utilizing Key Labs' innovative tools and platforms, businesses can significantly enhance their data labeling machine learning projects.
How Data Labeling Enhances Business Outcomes
Understanding how effective data labeling can impact business outcomes is crucial. Numerous industries are reaping the benefits of machine learning through well-annotated data:
1. Healthcare
In healthcare, correctly labeled data enables the development of predictive models that can help in diagnosing diseases early. Utilizing data annotation tools, healthcare professionals can train models that sift through vast medical records, significantly enhancing decision-making processes.
2. Retail
The retail sector benefits from machine learning applications in inventory management, customer personality profiling, and personalized marketing. With accurate labeling of consumer behavior data, stores can optimize stock levels and enhance customer experiences.
3. Autonomous Vehicles
Data labeling is crucial for developing self-driving technology. For autonomous vehicles to navigate safely, they must accurately recognize their environments—labels indicating pedestrian locations and traffic signals are vital for this technology’s success.
Best Practices for Data Labeling
Engaging in effective data labeling is essential for achieving the desired outcomes in machine learning. Here are some best practices:
- Define Your Guidelines: Establish clear guidelines for labelers to ensure consistency.
- Invest in Training: Comprehensive training programs for annotators can significantly enhance labeling quality.
- Implement Quality Checks: Regularly review labeled data to maintain high standards.
- Utilize Automated Tools: Leverage automated tools to speed up the labeling process without sacrificing quality.
By adhering to these best practices, businesses can foster an environment where data labeling leads to successful machine learning implementation.
The Future of Data Labeling in Machine Learning
The prevalence of machine learning continues to grow exponentially, and with it, the demand for effective data labeling. The future will be defined by:
- Increased Use of AI for Labeling: The integration of artificial intelligence will streamline the data labeling process.
- Enhanced Collaboration Tools: Future platforms will support better collaboration among data scientists, engineers, and business analysts.
- Focus on Ethical AI: There will be a growing emphasis on ethical considerations in AI, leading to the need for unbiased data labeling techniques.
The continued evolution of data labeling practices will play a significant role in shaping the future landscape of machine learning.
Conclusion
In conclusion, data labeling is not just a step in the machine learning process; it is vital to delivering accurate and actionable insights for businesses. With platforms like Key Labs leading the charge, organizations have the tools they need to harness the power of machine learning effectively.
As industries evolve towards a more data-driven future, mastering data labeling will become increasingly critical. Investing in high-quality data annotation practices not only enhances model performance but also drives innovation and growth opportunities across various sectors.